Inorganic crystals are a critical component of modern technologies. Their highly-ordered atomic structures provide them with unique chemical, electronic, magnetic, or optical properties that can be used in everything from batteries to solar panels, microchips to superconductors.
Crafting novel inorganic crystals in a lab — whether to enhance an existing technology or power a new one — is fairly straightforward, in theory. A researcher sets up the conditions, runs the procedure, and lets their failures inform how to tweak the conditions next time. Rinse and repeat until you have a new, stable material.
In practice, however, the process is fiendishly time-consuming. Traditional methods rely on trial-and-error guesswork that either tweaks a known crystalline structure or takes a shot in the dark. It can be expensive, take months, and, if things go wrong, leave researchers with little idea as to how or why.
According to the Materials Project, an open-access database founded at the Lawrence Berkeley National Laboratory, human experimentation has led to the discovery of around 20,000 inorganic crystals. Over the last decade, researchers have used computational methods to up that number to 48,000.
Enter DeepMind, Google’s AI research laboratory. Its researchers recently released the results of a new deep-learning AI designed to predict the potential structures of previously unknown inorganic crystals. And the results are centuries ahead of schedule.
Google’s crystal-making ball
DeepMind’s new AI is called the “Graph Networks for Materials Exploration” (or GNoME for short). As the name suggests, it is a graph neural network that operates by realizing the connections between data points through graphs.
GNoME trained on data available from the Materials Project and, using those 48,000 previously discovered inorganic crystals as a foundation, began constructing theoretical crystal structures. It created its predictions using one of two pipelines. The first pipeline, known as the “structural pipeline,” based its predictions on previously known crystal structures. The second pipeline, known as the “compositional pipeline,” took a more randomized approach to see what molecules it could squeeze together.
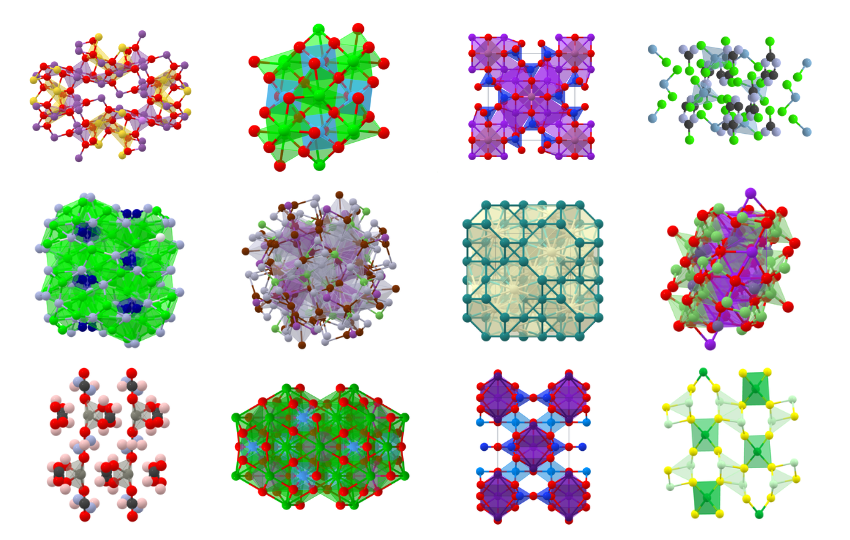
The AI then tested its predictions with “density functional theory” — a method used in chemistry and physics to calculate the structure of atoms. Whether the result was a failure or a success, it generated more training data that the AI could learn from. This, in turn, informed future pipeline predictions.
In essence, the AI’s pipelines and subsequent learning mirrors the human experimental approach outlined above. It just takes advantage of the AI’s processing power to perform the calculations at a much faster speed.
“Emphatically, unlike the case of language or vision, in materials science, we can continue to generate data and discover stable crystals, which can be reused to continue scaling up the model,” the researchers write.
All told, GNoME predicted 2.2 million new materials. Of those, around 380,000 are considered the most stable and will be prime candidates for synthesis moving forward. Examples of these potential inorganic crystals include layered, graphene-like compounds that may help develop advanced superconductors, and lithium-ion conductors that could improve battery performance.
“GNoME’s discovery of 2.2 million materials would be equivalent to about 800 years’ worth of knowledge and demonstrates an unprecedented scale and level of accuracy in predictions,” Amil Merchant and Ekin Dogus Cubuk, study authors and Google DeepMind researchers, add.
The research team published their findings in the peer-reviewed journal Nature. DeepMind will also contribute the 380,000 most stable materials to the Materials Project, where they will be freely available to researchers.
Autobots assemble!
GNoME’s predicted materials are theoretically stable, but few have been experimentally verified. To date, researchers have independently produced only 736 of them in a lab. While this does suggest the model’s predictions are accurate to a point, it also shows the long road leading to all 380,000 being experimentally fabricated, tested, and applied.
To close the gap, the Lawrence Berkeley National Laboratory tasked their new A-Lab, a type of experimental lab that combines AI and robotics for fully autonomous research, to craft 58 of the predicted materials.
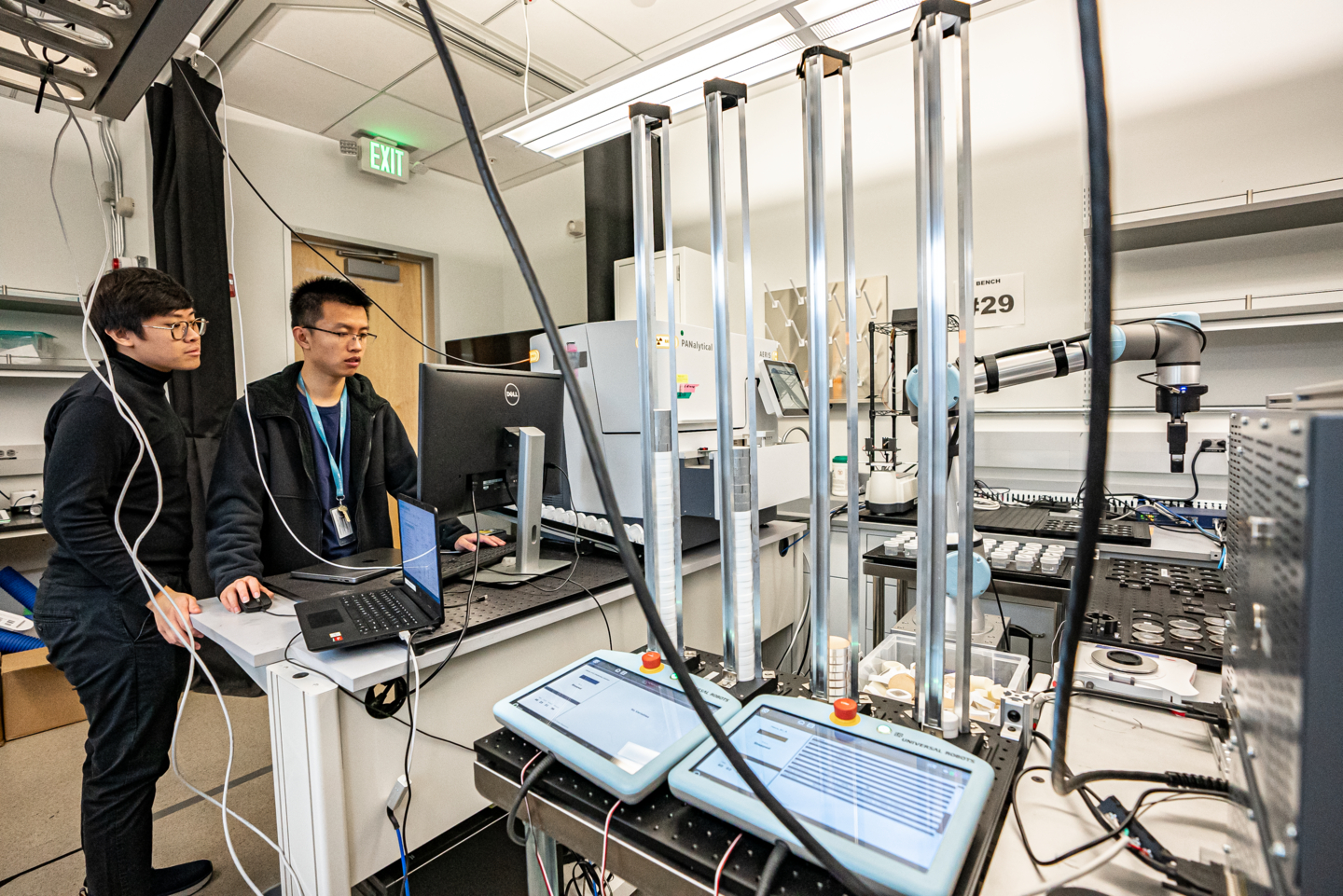
A-Lab is a closed-loop system, meaning it can make decisions about what to do next without human input. Once given its mission, it can select and mix the starting ingredients, inject admixtures during heating, prepare the final product, and extract it into an X-ray diffractometer for analysis. The lab’s AI will use that analysis to inform future attempts. And it can manage 50 to 100 times as many samples per day than a typical human researcher.
“Some people might compare our setup with manufacturing, where automation has been used for a long time. What I think is exciting here is we’ve adapted to a research environment, where we never know the outcome until the material is produced,” Yan Zeng, a staff scientist at A-Lab, says. “The whole setup is adaptive, so it can handle the changing research environment as opposed to always doing the same thing.”
Over a 17-day run, A-Lab says they successfully synthesized 41 of the 58 target materials. That’s more than two materials per day and a success rate of 71%. The NBNL researchers published their findings in another Nature paper*.
“With its high success rate in validating predicted materials, the A-Lab showcases the collective power of ab initio [from the beginning] computations, machine-learning algorithms, accumulated historical knowledge, and automation in experimental research,” the researchers write in the study.
The researchers are also looking into why the remaining 17 inorganic crystals didn’t pan out. In some cases, it may be that GNoME’s predictions contained inaccuracies. For others, it could be that broadening A-Lab’s decision-making and active-learning algorithms could yield more positive results. In two cases, retrying with human intervention led to a successful synthesis.
We have to create new materials if we are going to address the global environmental and climate challenges.
Kristin Persson
As such, GNoME has given A-Labs and human-run research facilities around the world much to work with for the foreseeable future.
“This is what I set out to do with the Materials Project: To not only make the data that I produced free and available to accelerate materials design for the world, but also to teach the world what computations can do for you. They can scan large spaces for new compounds and properties more efficiently and rapidly than experiments alone can,” Kristin Persson, the founder and director of the Materials Project, said.
He added: “We have to create new materials if we are going to address the global environmental and climate challenges. With innovation in materials, we can potentially develop recyclable plastics, harness waste energy, make better batteries, and build cheaper solar panels that last longer, among many other things.”
* Author’s note: Since the A-Lab paper’s publication in Nature, some scientists have called for its retraction. They claim the A-lab didn’t properly analyze the created materials and therefore could not make reliable identifications. You can read about the ensuing debate here.