The first IBM Personal Computer debuted in August 1981. It was also the first computer to use Microsoft’s MS-DOS operating system (technically, a nearly identical copy known as IBM PC DOS, also known as PC DOS or IBM DOS). At the time, Unix held a roughly 55% share of the market for operating systems running on personally owned devices; the rest was split between Xerox’s Alto (launched in 1973 for proto-PCs), CP/M (1974, for microcomputers), TRSDOS (1977, also microcomputers), Commodore KERNEL (1977, home computers), Atari DOS (1979, home computers), Apple’s DOS (1978, microcomputers), and SOS (1980, microcomputers). By 1985, MS-DOS had amassed more than 50% market share. By 1989, it held just under 90%.
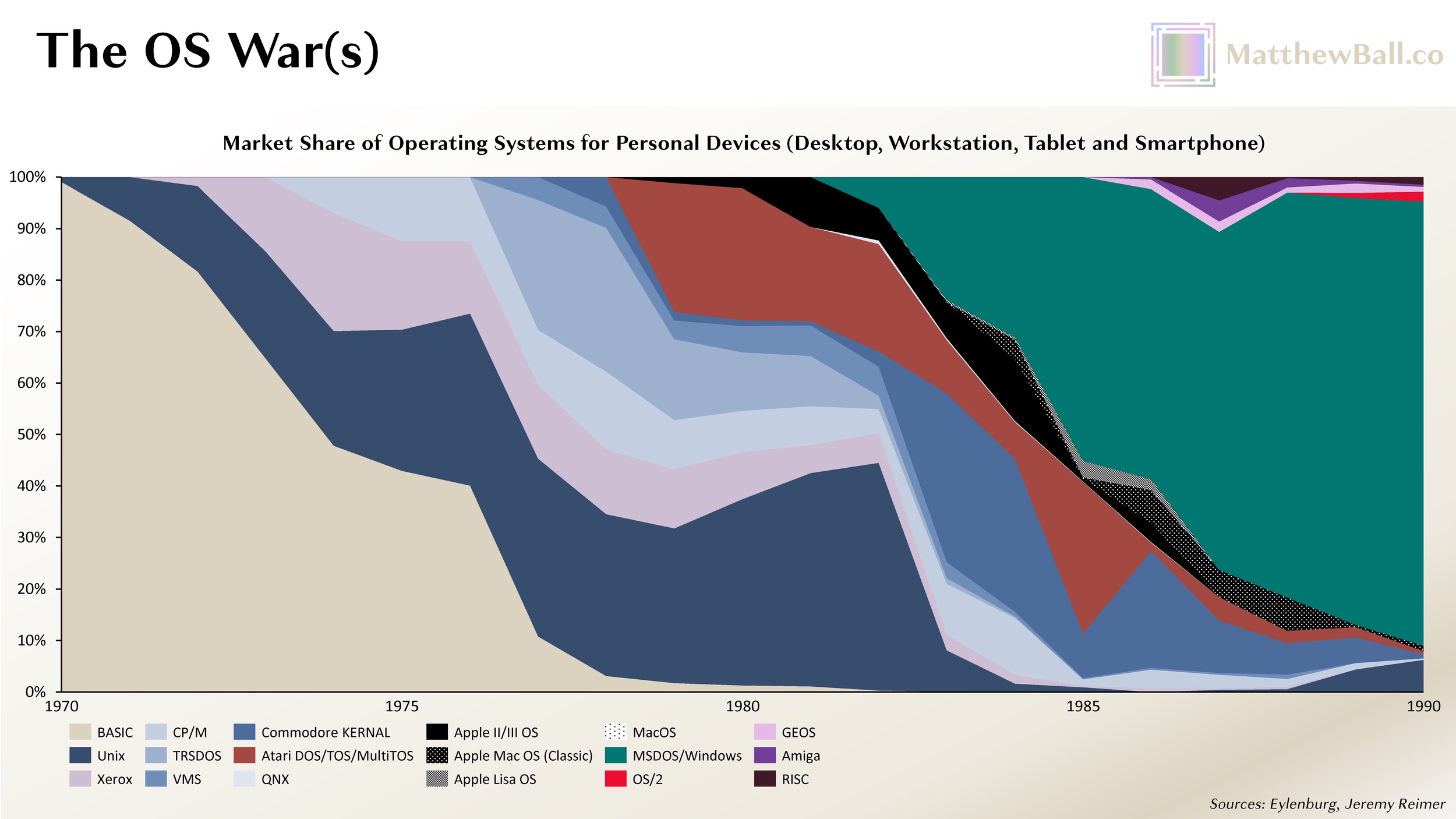
Despite Microsoft’s success throughout the 1980s, the company’s founder and CEO, Bill Gates, suspected that DOS was approaching its end. Each year, there were more people who owned a PC, even more who used one as part of work, and leisure usage was growing rapidly, as was the number of PC developers. These changes were great for the market, and Microsoft was a primary beneficiary of this market, but these changes changed the market, too, which could jeopardize Microsoft’s position. There was also growing evidence of competitive and structural changes in the marketplace. Apple’s line of graphical user interfaces (GUI) computers, which debuted with the 1983 Lisa PC, were increasingly popular and served as an obvious contrast to the command-line interfaces of DOS. In 1984, MIT had kicked off a project to build the Window System for Unix and Unix-like OS. In 1985, IBM had begun development of OS/2, which Microsoft signed on to co-develop, but in contrast to IBM DOS, OS/2 was spearheaded by IBM, not Microsoft, and designed to sell IBM PCs and hardware, not foster the PC hardware ecosystem or Microsoft’s Windows. In 1986, a group that spanned Sun, AT&T, and Xerox (which had pioneered the GUI interface) began working on a GUI specification of Unix (OPEN LOOK, which later progressed into OpenWindows) that would make the OS more consumer-friendly. In 1988, a similar collective including IBM, HP, Compaq, and DEC formed to build Digital Unix (a.k.a. Tru64 UNIX).
Taken together, it was not hard to predict that platform-level changes might soon occur. If so, secondary and tertiary changes were inevitable, too. In other words, Microsoft faced not just the prospect of new competitors, some of which were current licensors, but also potential disruption in its OS licensing business model, all of which threatened its growth, investment, and product strategy and might alter industry profit pools as well.
To manage this uncertainty, Microsoft undertook a portfolio of bets throughout the 1980s and early 1990s that were often competitive with one another (or had competing premises) but collectively replicated the diversity, unpredictability, and dynamism of the market at large, thereby maximizing Microsoft’s odds of success in any future state. These bets were roughly as follows:
- Continue development of MS-DOS;
- Collaborate with the many companies working on UNIX, namely through the ongoing development of its Xenix version of Unix (1980–89);
- Commence major investment(s) in Windows, a GUI OS (development started 1983, with Windows 1.0 shipping in 1985 and 3.0 in 1991)
- Form partnership with IBM to develop OS/2 (1985–);
- Purchase 20% stake in Santa Cruz Operation, the largest seller of Unix systems on PCs (1989); and
- Develop suite of applications (namely Microsoft Office, 1990–) that could operate across operating systems which Microsoft might have no ownership (or influence) over
While Microsoft had many bets, it still had its preferred one: Winning the personal computing market, via a licensed OS. They wanted the OS that won out to be Windows. As Microsoft had been successful with this core endeavor, it also could have proceeded with that sole bet.
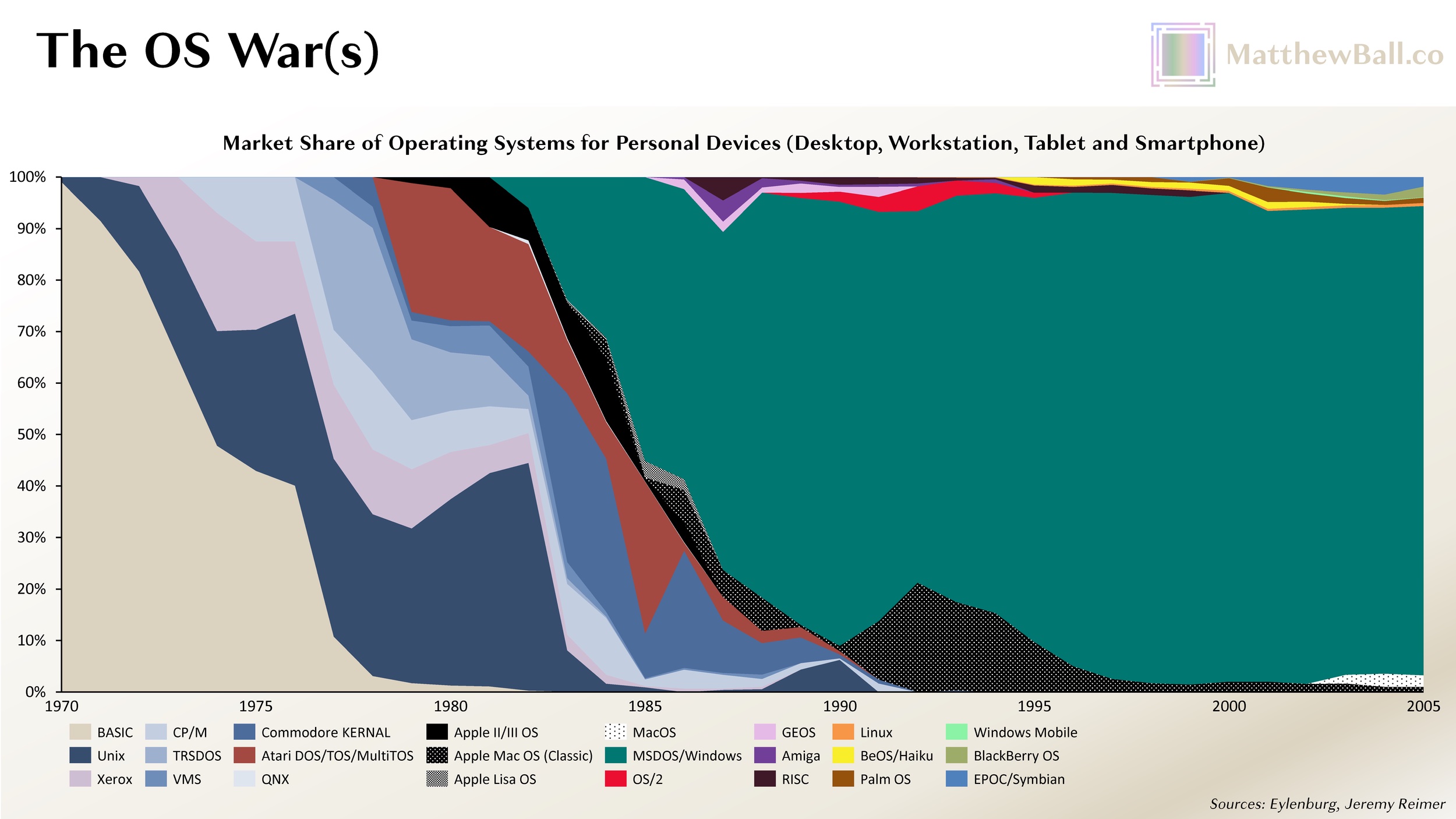
Yet a live-or-die bet wasn’t necessary, and indeed, some of those failed bets failed precisely because Windows 3.0, which launched in 1990, was so successful. One such example is OS/2. This jointly developed OS was always challenged by the dueling priorities of IBM and Microsoft, but following the breakout of Windows 3.0, the partnership between the two companies became impossible to sustain. IBM took sole ownership in 1992 (the final release was in 2001). By 1993, the Unix ecosystem had determined that a fully unified Unix was needed to combat Windows, prompting the Common Open Software Environment initiative, or COSE (founded by the Santa Cruz Operation, Univel, Unix Systems Laboratories, Sun, HP, and IBM). These hopes were dashed by the blockbuster Windows 95, which Santa Cruz, HP, and IBM had little choice but to support, especially as rivals such as Dell picked up market share. It’s likely that Microsoft also had picked up extensive knowledge from its various bets, such as the rationale behind various technical and interface-related decisions across O/2, Unix, and so forth, and used this knowledge to strengthen relationships with key industry partners, most notably those who manufactured and distributed PCs.
Even as Windows secured the market in the early 1990s, Microsoft remained paranoid about the right product offering. Prior to the launch of Windows 95, the company released Microsoft Bob, which was intended to be an even more consumer-friendly GUI for novice computer users, but it failed miserably and was quickly killed the following year.
In 1995, Gates wrote his famous “Internet Tidal Wave memo,” in which he argued that the Internet was not just a critical new frontier for Microsoft, but one that might empower the company’s OS competitors or even displace the role of the OS altogether:
I assign the Internet the highest level of importance… I want to make clear that our focus on the Internet is crucial to every part of our business… The Internet is the most important single development to come along since the IBM PC was introduced in 1981. It is even more important than the arrival of the graphical user interface (GUI). The PC analogy is apt for many reasons. The PC wasn’t perfect. Aspects of the PC were arbitrary or even poor. However a phenomena grew up around the IBM PC that made it a key element of everything that would happen for the next 15 years. Companies that tried to fight the PC standard often had good reasons for doing so but they failed because the phenomena overcame any weaknesses that resisters identified… IBM [also] includes Internet connection through its network in OS/2 and promotes that as a key feature. Some competitors have a much deeper involvement in the Internet than Microsoft. All UNIX vendors are benefiting from the Internet since the default server is still a UNIX box and not Windows… Sun has exploited this quite effectively… and [is] very involved in evolving the Internet to stay away from Microsoft… [Moreover], a new competitor “born” on the Internet is Netscape.
Gates’s memo led to a flood of investment in the company’s digital efforts, from Internet Explorer (1995) to the MSN portal and search engine (1995), the $400 million acquisition of Hotmail (1997; $760MM in 2023 dollars), Messenger (1999); the list goes on. The company also remained diversified in its traditional OS bets, purchasing a 5% stake in Apple in 1997 for $150MM; the deal also involved Apple making Internet Explorer the Mac’s default browser).
The waves roll on
As we review Microsoft’s parallel bets in 2023, a few takeaways are clear. Eventually, Microsoft lost most of the market in consumer internet services, such as search and web portals, email, messaging, and identity. Several of these markets are more lucrative than operating system licensing. While Microsoft successfully boxed out Unix, Linux, an open-source OS that launched in 1991, has thrived (though differently than its creator once imagined). Today, more than 95% of the 150–200 million operating servers run Linux, and Android, the most used operating system in history, is also based on the OS. Microsoft’s modern-day strength in productivity software and other horizontal/cross-platform technology, such as Azure, has also enabled the company to thrive even after its OS was displaced—although this displacement was not at the hands of PC-related competitors in the ’80s or ’90s but rather two mobile competitors of the 2000s and 2010s, iOS and Android. It also turned out that mobile computers would become more than two-thirds of the market, capping Microsoft’s share of the total OS market to a third at best.
Crucially, Microsoft’s displacement in the mobile market resulted from undiversified thesis errors. In his infamous January 2007 CNBC interview, Microsoft CEO Steve Ballmer laughs at the prospects of the just-announced iPhone, citing its high price and lack of a keyboard. Popular recollections of this interview typically ends here, but Ballmer’s full report is more generous, saying “it may sell very well,” after which he explains why Microsoft’s strategy is superior. It was the foundational theses of Microsoft’s mobile strategy, which rested on concepts that were shared by most of the proto-smartphone, most notably BlackBerry and Palm, that doomed the company’s efforts. Smartphones should be $100–$200, not $500 or more; they should focus on business users not consumers; they should feature a keyboard; data usage should be minimized so as to protect scarce network bandwidth; batteries should last for days, not hours; fall damage should be minimal, rather than device-wrecking. Though these bets were likely “right” in the late 1990s and early 2000s, they proved wrong over time.
Microsoft also continued to apply its PC-era business model, rather than diversify or really even test other hypotheses. When MS-DOS debuted, there were already a handful of computer manufacturers, most of which supported multiple operating systems (and some consumers chose to install their own at a later time, anyway). Microsoft grew its share of the market not by competing with these OEMs, by producing its own PC, or by partnering exclusively with IBM but by licensing Windows for $50–$100 to any or all manufacturers. For the most part, Apple limited its OS to its own hardware, the first iteration of which debuted in 1976 (five years before the IBM PC or MS-DOS). Some in the tech community believed the company produced better “PCs,” but its vertically integrated approach led to higher prices and constrained distribution, and the company struggled mightily to overcome the large and incredibly developed PC and Windows ecosystem. Microsoft, of course, knew that there were potential benefits from producing its own first-party hardware, but doing so would mean competing with its many partners, most of which could (and would) renew their own OS investments in response, or worse, adopt a would-be rival to Windows, such as Unix.
When it came to the early “smartphones” of the late 1990s and early 2000s, Microsoft’s approach (Windows Compact and Windows Mobile) was similar to its PC strategy, though it charged a more modest $10–$25 per device. The challenge here was that mobile computers were comparatively harder to build than PCs due to the vastly different constraints on size, power usage, heat generation, and the like. But in the mobile form factor, which was so nascent that fewer than 60MM devices had been sold over fifteen years, Apple’s vertically integrated approach led to a substantially better device. Unlike Microsoft, Apple built a truly mobile-native OS, rather than porting over most of the design principles of its Mac OS (Windows Mobile literally had a taskbar and Start menu button). The result was a device that totally outclassed Windows-based smartphones and effectively downgraded the other would-be smartphones to proto-smartphones.
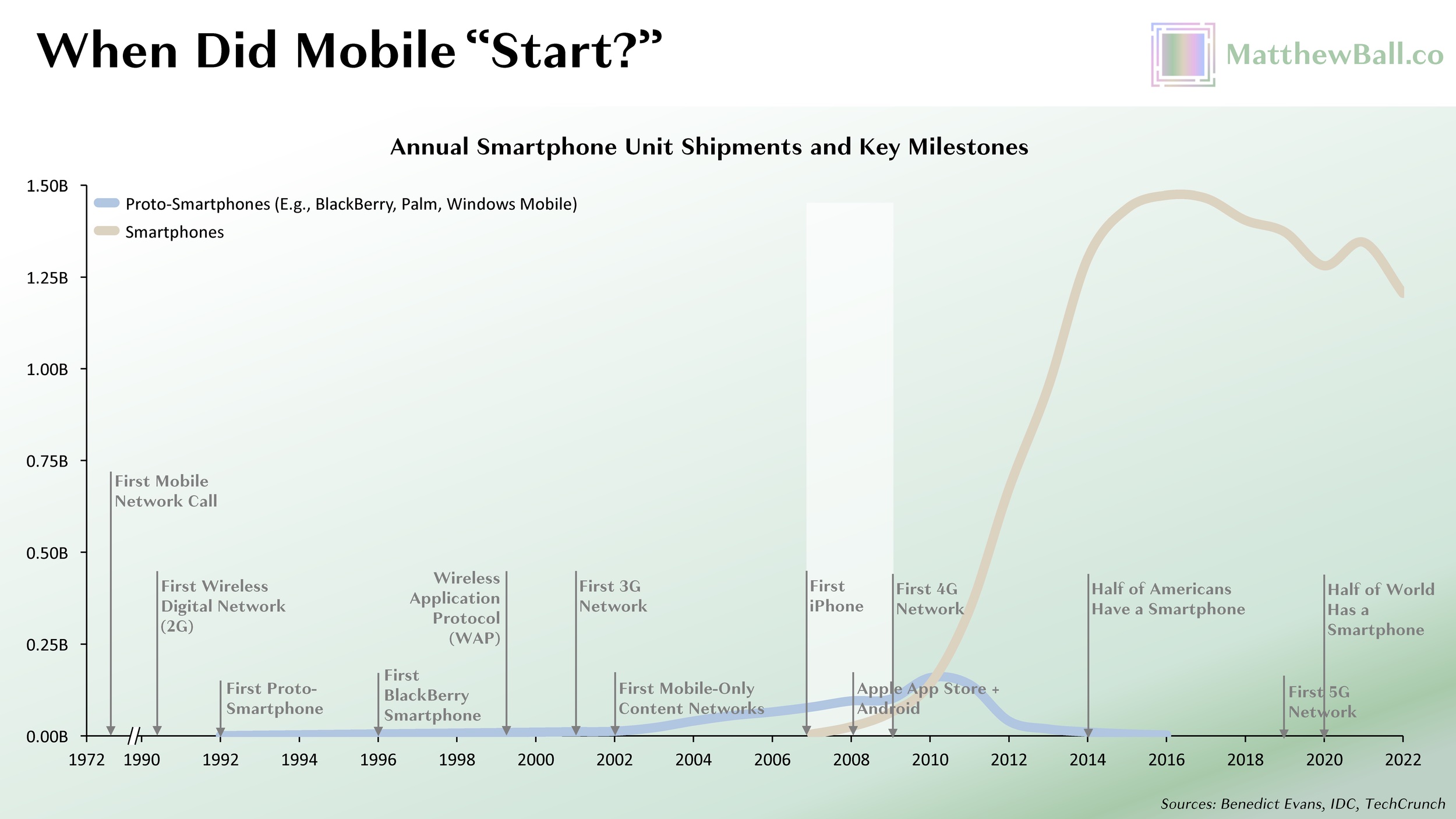
Independent smartphone manufacturers rushed to catch-up to Apple’s hardware designs, and were somewhat successful, while also differentiating on other designed-related features (e.g., offering better cameras or larger screens). However, these OEMs fell well short on the OS and ecosystem side, thereby preserving the PC-era opportunity for an independent OS provider. Here however, Microsoft’s Windows Mobile lost to Google’s Android, which was not just a more modern OS (mobile-native, touch- and consumer-focused, and so on) but free to device makers. In fact, Google offered OEMs (and wireless carriers) a share of Android-related search and Google Play app store revenues. In effect, the OS business model had inverted from direct monetization (Microsoft’s bread and butter) to the sale of profitable hardware (Apple’s main business line and one in which Microsoft lacked any business at all) and software/services bundles (where Google thrived and Apple was rapidly growing). By the time Microsoft pivoted, launching its free-to-license Windows Phone OS in 2010 and an exclusive partnership with Nokia a year later (Microsoft ended up buying it in 2014), it was too late. Had Microsoft been earlier in its shift, or just partly wrong about smartphones when the iPhone launched, its mobile OS might have survived. (Incidentally, MacOS, iOS, and Android are all UNIX-based).
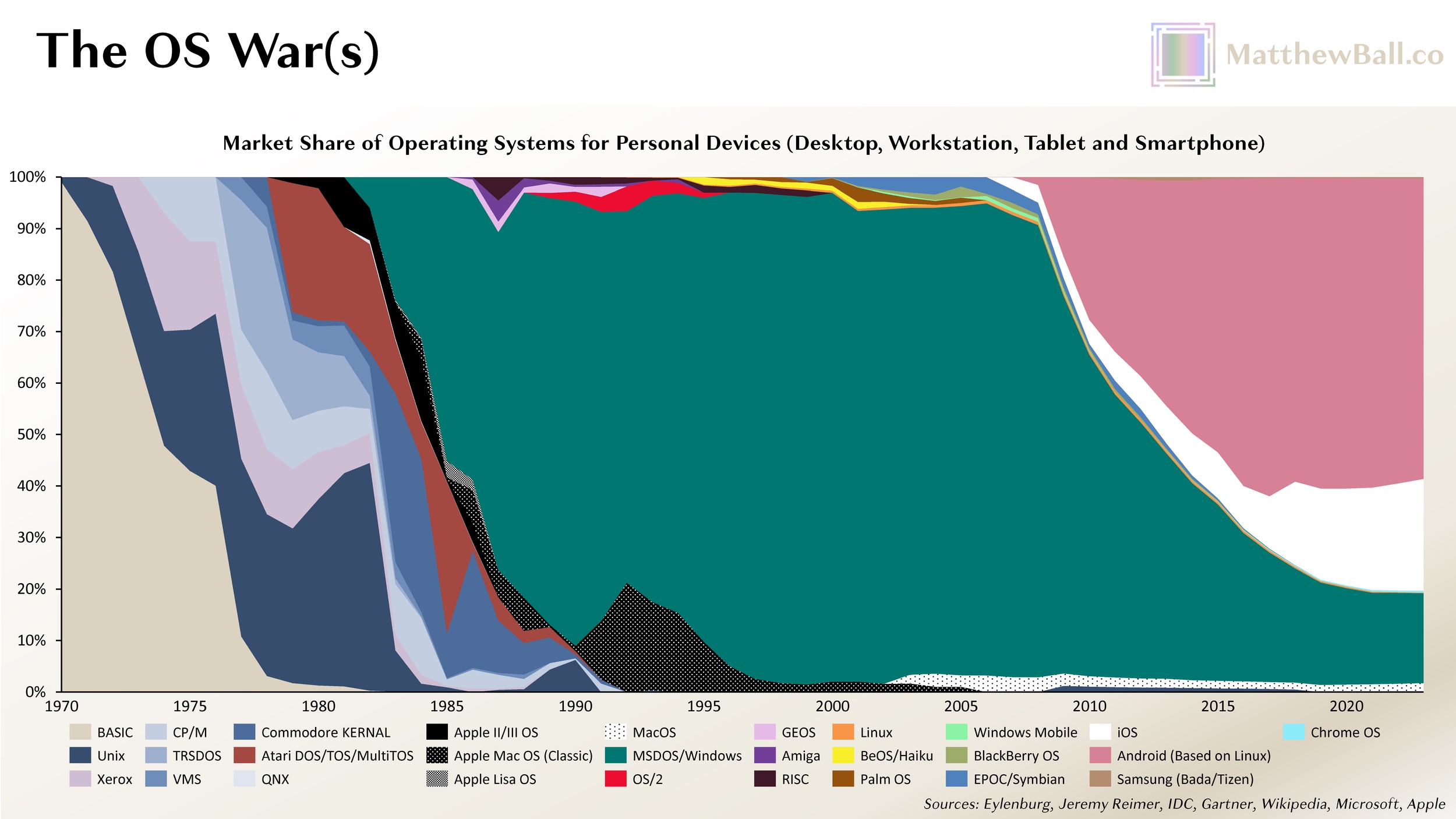
Smart, confident but seemingly confused
I think a lot about parallel bets when it comes to AI, a field with such obvious, diverse, and immense potential that also faces great uncertainty around technology, business model, timing, cost, and implementation. Few debate that the emergence of the “AI Era” could produce a new tech titan, just as few debate that it could crush (or outright destroy) one that today seems indefatigable. Perhaps it is no surprise, then, that Microsoft is deploying a wide array of strategies to manage various uncertainties, many of which compete with one another or otherwise seem to conflict with the company’s overall AI strategies and investments.
Though Microsoft Research was not formally established until 1991, Microsoft’s market-leading investments into AI began not long after Gates founded the company in 1975. The results of these investments were similarly prompt. Microsoft’s first text processor, Word 1.0 for DOS, launched in 1983 and differentiated from market leaders and other would-be competitors through the integration of “Spell Check.” At the time, MicroPro’s WordStar required users to launch a separate spell-checking program, while WordPerfect lacked the capability altogether. Though “Spell Check” falls short of modern definitions of AI (more on this later), Microsoft slowly grew from basic probability matching (e.g., when a word didn’t match a known word in Microsoft Word’s database, its letters and their sequence would be checked for the best fit against that database) to a more complex system that could recognize words that were spelled correctly but didn’t suit a sentence (e.g., homophones), recommendations that were based on the rest of a sentence rather than just the word itself (e.g., “lichense” might be closer to “lichens,” but “license” better fits the sentence overall) and then eventually learning from a specific user’s errors as well as those of all users, too. Along the way, Microsoft also added grammar check, too, which started with basic corrections (e.g., tense and number agreement) before moving to stylistic suggestions such as clarity and concision. Microsoft’s Clippy, which debuted in 1996, was not a popular product, but it was a pioneer in digital assistants and using Natural Language Processing to infer what users were attempting to do in order to provide advance recommendations. Though Clippy was removed from Microsoft’s Office Suite in 2007, turned off by default in Office XP, and ignored or disabled by users earlier still, the very investments behind Clippy powered Microsoft’s far more successful features, such as predictive data entry (e.g., drag and fill), inconsistent formula warnings), and recommended analysis in Excel, as well as formatting and template suggestions in Word, Mail, and PowerPoint, and later, Microsoft Dynamics CRM.
By the 2020s, it’s likely that no company had invested more in AI than Microsoft. Still, the company’s executives had begun to fear that it was falling behind—and that just as was the case in smartphones, competitors might quickly surge beyond them. In a series of 2019 emails (which were released in 2024 as part of the Department of Justice’s antitrust case against Google), Microsoft’s CTO (Kevin Scott), CFO (Amy Hood), CEO (Satya Nadella), and founder (Gates) express in detail this very fear. Scott, who initiates the thread, writes that he had previously been “highly dismissive of [competitor’s] efforts. . .. [but] that was a mistake. . . [I am] very, very worried.” Scott’s epiphany was prompted by work to better understand how Google was bringing together its largely disconnected and sometimes rivalrous internal AI initiatives—i.e., its parallel bets, of which the most notable are Google Brain and Deep Mind—as well as the advances of OpenAI, which had been founded only four years earlier and had roughly 100 employees.
“The thing that’s interesting about what [they] are doing is the scale of their ambition, and how that ambition is driving everywhere from datacenter design to compute silicon to networks and distributed systems architectures to numerical optimizers, compilers, programming frameworks, and the high-level abstractions that model developers have at their disposal,” wrote Scott. “When they took all of the infrastructure that they had built to build [natural language processing] models that we couldn’t easily replicate, I started to take things more seriously. And as I dug in to try to understand where all of the capability gaps were between Google and us for model training, I got very, very worried. We have very smart [machine learning] people in Bing, in the vision team, and in the speech team. But the core deep learning teams within each of these bigger teams are very small, and their ambitions have also been constrained…. and we are multiple years behind the competition.”
In response to Scott’s email, Nadella replied, “Very good email that explains why I want us to do this [deal] and also why we will then ensure our infra folks execute.” The deal in question was a $1 billion investment into OpenAI, which had privately released its GPT-1 model only a year earlier (2018) that was based on the transformer model that had been initially proposed by Google Brain and Google Research barely a year before that (2017). Before shifting to transformers, OpenAI had invested in many alternative approaches to AI, including evolutionary algorithms and Long Short-Term Memory (LSTM). As part of its investment, Microsoft also granted OpenAI with free access to its Bing search database, which the startup then used to train and improve its generative pre-trained transformer (GPT) models.
Between 2020 and 2022, Microsoft invested another $2 billion into OpenAI while continuing to scale its internal teams and outside investments. In 2021, Microsoft announced its second-largest acquisition ever, spending $20 billion to acquire Nuance Communications, a thirty-year-old AI pioneer with particular strength in natural language processing and speech recognition (Nuance’s engine was reportedly at the foundation of Apple’s Siri) as well as healthcare-specific AI.
Despite Microsoft’s acquisition of Nuance, internal investments in AI, and its chief officer’s hopes that its OpenAI investment would help inspire Microsoft’s internal groups to scale up, catch up, and “execute,” Nadella remained dissatisfied. By December 2022, Nadella had reportedly concluded that the models developed by OpenAI’s 250-person team (GPT-3.5 and a pre-release version of GPT-4) had surpassed those of Microsoft Research so thoroughly that he asked division’s chief, “Why do we have Microsoft Research at all?” Barely a month later, Microsoft invested $10 billion into OpenAI in exchange for a (de facto) 49% ownership stake in the company as well as 75% of profits until that $10 billion is recouped (and 49% thereafter, to an unknown cap), in exchange for broad rights to incorporate its technologies into Microsoft’s own offerings. And then Microsoft quickly went to work.
Only two weeks after Microsoft’s OpenAI investment, the companies announced the “New Bing,” with Microsoft proudly boasting it was powered by OpenAI’s GPT-4 and even co-branding its search engine as “BingChat with ChatGPT-4” or even “Bing Powered by ChatGPT’s GPT-4.” That same month Microsoft announced Copilot, a GPT-powered chatbot that would be integrated across its Office Suite and other tools, including Microsoft 365 and, most importantly, GitHub. Three months later, Microsoft announced the end of Cortana, the virtual assistant launched by the company in 2014 following the release of Apple’s Siri (2011), Google’s Now/Assistant (2012), and Alexa (a month earlier in 2014).
Given Microsoft’s highly marketed deployment of OpenAI-branded products as well as its ongoing deal to provide search data to OpenAI and its near-majority stake in the company and even greater rights to OpenAI’s foreseeable profits, one might assume that Microsoft had picked its “One Big Bet.” Not so! Although Microsoft makes extensive use of ChatGPT’s branding, its products technically run on Microsoft’s own Prometheus model. Prometheus is built on GPT-4’s foundational large language model but was subsequently fine-tuned by Microsoft using both supervised and reinforcement learning techniques. As such, Microsoft not only owns the end-user of when they use “Powered by GPT” products, as well as their related engagement/query data, they also operate part of the model itself and can, if they so choose, begin to substitute OpenAI’s products out over time. Indeed, The Information reports that this is Microsoft’s express goal—starting with a model that “may not perform as well . . . [but] costs far less to operate,” as Microsoft covers all of the compute-related costs from resolving a GPT-powered query or prompt. Microsoft’s GPT-powered (and often branded) products are also in obvious competition with OpenAI’s own consumer-facing GPT-3.5, GPT-4, GPT-4o, Codex, et al.; a user who picks the latter to resolve a specific query or answer a specific prompt has no need for Microsoft to do the same.
When Meta open-sourced its Llama model—a move that threatened OpenAI’s business model and thus also Microsoft’s potential royalties from said business model—Meta did so in headline partnership with Microsoft, which Meta dubbed its “preferred partner.” In February 2024, Microsoft invested in Mistral, another transformed-based AI leader, at a $2.5B valuation. Notably, Mistral was founded two months after Microsoft struck its groundbreaking $10B OpenAI investment; notably, like Meta, Mistral produces open-source models. A month later, Microsoft struck a $650-million deal with Inflection AI, a $4 billion-valued startup founded by Reid Hoffman, a co-founder of LinkedIn, and Mustafa Suleyman, who had previously co-founded DeepMind, acquired by Google in 2014. As part of this deal, Microsoft not only licensed most of Inflection’s models, it also took on most of Inflection AI’s staff, while Suleyman became CEO of the newly announced Microsoft AI, which reported directly to Nadella. Karén Simonyan, another co-founder of Inflection AI, also joined Microsoft as “Chief Scientist of Microsoft AI.” There are some reports that Microsoft has made its Bing search database available to other AI startups and companies interested in building their own foundation models.
Microsoft’s relentless diversification away from OpenAI is a easier to understand when placed in the context of OpenAI’s own efforts. Take search. The day Microsoft launched the New Bing, Nadella boasted to The Verge, “I hope that, with our innovation, [Google] will definitely want to come out and show that they can dance. And I want people to know that we made them dance.” The company also estimated that every 1% in market share that New Bing might acquire would be worth as much as $2 billion annually. Yet only a month after the release of New Bing, DuckDuckGo, the 6th-most-used search engine globally with 0.55% (compared to Bing’s 2nd place position with 2.75%), announced its ChatGPT-powered DuckAssist service. According to reports, OpenAI has recently struck a deal to power a new version of Apple’s Siri (which, incidentally, defaults to Google for traditional search!). OpenAI is also rumored to be developing its own search engine, and recently hired Shivakumar Venkataraman, a 21-year Google veteran who ran Google Search’s ad business. Microsoft has touted its GPT-powered Copilot integration in its Dynamics 365 CRM offering, yet that same product’s chief competitor, Salesforce, has also licensed ChatGPT in order to build its similar EinsteinGPT feature. In May 2024, OpenAI announced it had developed a desktop application for its suite of AI products—but it was Mac-only, with the company’s CTO blandly maintaining that the company is “just prioritizing where our users are.”
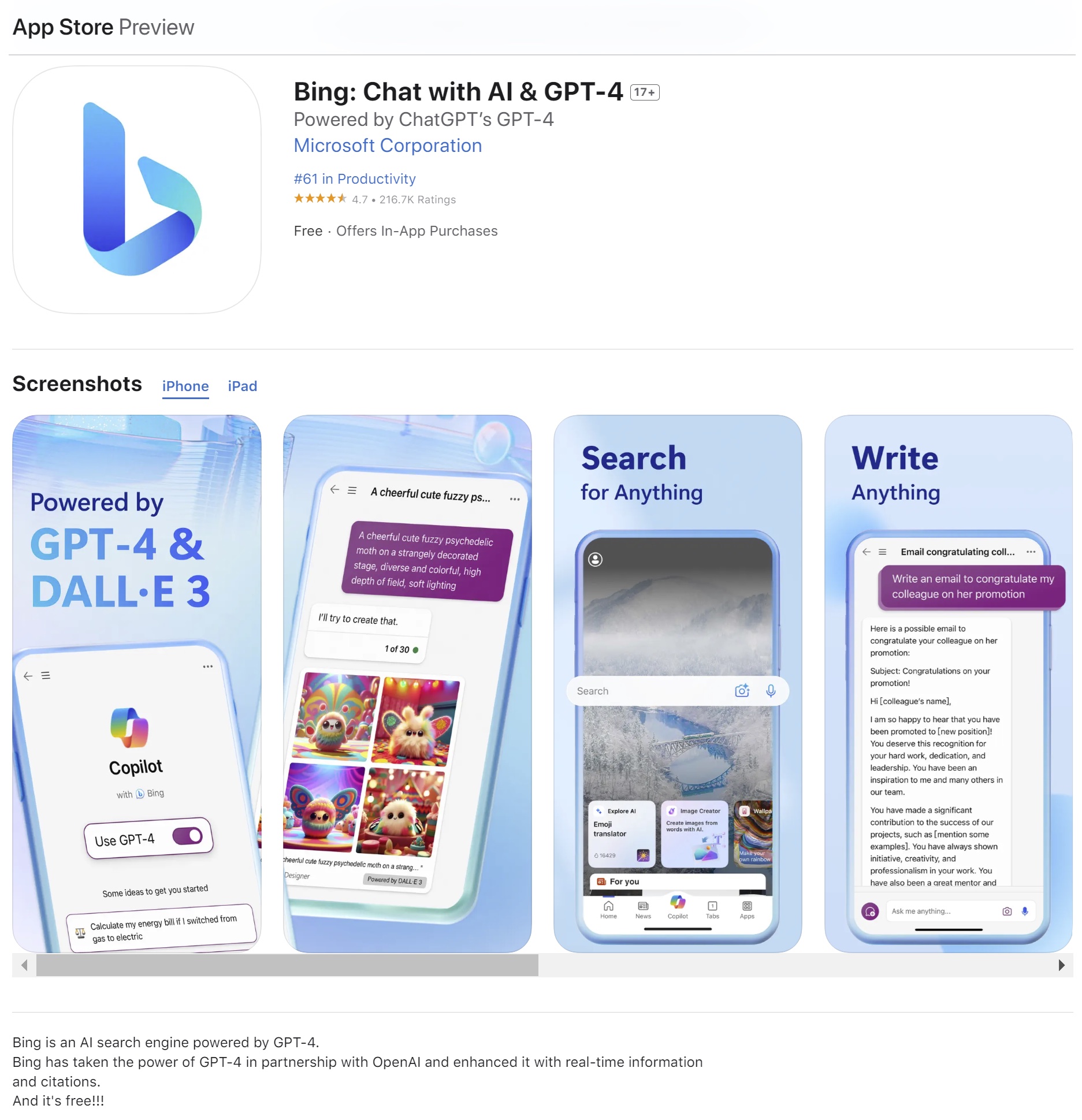
The most threatening limits of Microsoft’s influence over and benefits from OpenAI are deeper beneath the surface. Even with its enormous stake in the company, Microsoft was not notified that OpenAI’s CEO was under (quasi?) investigation by its board for a lack of candor— (apparently?) —nor that he was due to be terminated. And while Microsoft has broad rights to integrate OpenAI’s products into their own, these rights do not extend to any systems the OpenAI board determines has “attained” artificial general intelligence (AGI), which would also make the “legacy” systems for which Microsoft still had rights rather obsolete.
And fundamentally, Microsoft’s competition with OpenAI’s direct-to-consumer products like ChatGPT or its integration into third-party services, such as Einstein GPT, threatens more than just the odd point of market share here and there. Microsoft understands that multimodal LLMs—which ChatGPT defines as “AI systems capable of understanding and generating content across different types of data, such as text, images, and audio, enabling them to process and integrate information from multiple sensory modalities”—can replace existing software interfaces entirely, rather than just diverting various queries, prompts, or functions or otherwise sitting alongside existing interfaces. And the interface layer tends to be the most profitable part of the digital value chain. It’s for this reason that Microsoft chooses to control the “reinforcement learning from human feedback” data in Prometheus, rather than relay it to OpenAI (or other partners) and/or use ChatGPT directly. It’s also why AI is now being shoved into anything that users can touch/see/use (at Microsoft and elsewhere). The crucial importance of RLHF is what makes AI zero-sum—and why a growing many believe Microsoft’s OpenAI investment/partnership to be both strategically brilliant and bound to erode (if not collapse outright).
Parallel lessons
Parallel bet strategies have several core advantages, from increasing corporate optionality (especially when it comes to acquisitions) to covering strategic bases, maximizing learnings, and (potentially) neutralizing (or at least moderating the risk of) any potential competitors. But there are costs. More money tends to be spent, but each bet tends to receive less funding than they might have under a more focused approach, which can constrain the would-be “winners.” Overseeing many bets can also lead to mixed signals on what is (and is not) the “right bet” and harm internal morale. Few teams feel good about competing against their colleagues. Worse still, these teams typically hate when their corporate parent funds external competitors that, with a little more funding and support, they might been able to beat but now threaten to put them out of a job.
In the context of Microsoft, it’s not hard to “what if” its parallel bets strategy. What if Microsoft had bought 49% of OpenAI earlier, if not the entire company, rather than hedging on the startup and other internal investments? Or at least formed a (more exclusive) commercial deal around the time of GPT-2 or GPT-3, back when OpenAI’s lead was more modest and its leverage similarly smaller, too? Perhaps with more money and attention and a longer competitive runway, Microsoft’s internal efforts might have surpassed those of OpenAI, rather than helped to make it the market leader. (Incidentally, the Motorola ROKR, also known as the “iTunes Phone,” was a parallel bet of both Motorola and Apple but was a failure for the former and helped drive and accelerate the latter’s iPhone initiative, which then helped destroy Motorola’s market share.)
There are, however, a number of timing-related flaws with those “what ifs.” The sudden maturation of transformer models was not predictable, for example, nor was the fact that OpenAI, rather than Cohere or Anthropic or Hugging Face, would be the market leader. OpenAI might not remain the market leader, and transformer models may eventually be replaced, too. And though powerful, transformer models address only some AI use cases.
Microsoft’s approach also contrasts with that of Amazon. Beginning in 2012, the company bet that the era of the “smart assistant” had begun. Amazon was not alone in that bet; Apple had shipped Siri with iOS a year earlier, with Google deploying its Google Now eight months later. Unlike Apple and Google, Amazon lacked a smartphone, and so the company instead focused on building a suite of lightweight devices that could be placed throughout the home and office and called upon at any moment. By 2016, the Alexa device was shipping tens of millions of units per year, with Amazon founder and CEO Jeff Bezos speaking openly of his hope that it would become the fourth pillar of the company, sitting alongside Amazon Marketplace, Amazon Prime, and Amazon Web Services. By 2020, lifetime device sales were in the hundreds of millions, an order of magnitude higher than those of Google’s Home/Nest devices, with Apple’s competitor, the HomePod, flunking out of the market. Amazon also began to unlock the Alexa software from its first-party hardware, deploying it widely in vehicles, such as those of Ford and Toyota, and even quasi-rivalrous speaker systems like Sonos.
Though Alexa’s topline performance suggested it was or at least could be the winner in “consumer AI,” the platform struggled to overcome core engagement problems. For example, the average consumer didn’t use Alexa very much—and when they did, queries were rudimentary (“What time is it?,” “Set an alarm for 2:05PM,” “Will it rain today?,” “Play Dua Lipa,” etc.). Few developers had produced Alexa apps (“Skills”), and even fewer integrated the platform (or its device) into their products. The result was a device that sold well but generated only abstract value to Amazon—and, if the reports are to be believed, tens of billions in losses. When Amazon began its layoffs in 2022, the Alexa division, which had an estimated 10,000–15,000 employees, was disproportionately affected. As transformer models took off toward the end of 2022, the optics of Amazon’s investments worsened as it became clear its costly bet had been wrong—at least for now. The Information has since reported that Amazon had planned to launch its own transformer LLM (“Bedrock”) in November of 2022 but shelved it after seeing ChatGPT and also that the company had passed on various product partnership and equity proposals from OpenAI, Anthropic, and Cohere dating as far back to 2018.
Throughout 2023, Amazon has reset its AI strategy. Dave Limp, the head of Amazon’s Alexa division, announced he was “retiring.” Amazon repurposed its Bedrock name to launch an AWS marketplace for third-party AI solutions and models, with the goal that AWS customers would run these products on AWS compute. In October 2023, Amazon announced a $1.25 billion investment in Anthropic, which had raised several hundred million dollars from Google across two fundraising rounds in January and May of that same year. As part of its investment, Amazon announced a “framework” through which it might invest another $2.75 billion into the company. A week later, Google responded with a $2 billion follow-on investment in Anthropic. Unsurprisingly, Amazon continues to develop its own foundational models, too.
Breakfast and dinner
Parallel bets strategies are best suited to (1) cash-rich companies . . . that are (2) pursuing “must-win” categories” . . . in which (3) their assets and strategies are a good fit . . . but (4) may not be configured correctly . . . and (5) there is a high rate of change . . . and (6) many uncertainties . . . and (7) many players . . . with (8) progress often occurring out of sight. Deployed correctly, a company can cover all of the bases while also neutralizing the existential threat of a new competitor. Parallel bets are therefore likely the right strategy generally for “Big Tech” and during this phase of AI, during which there are many unresolved and interconnected hypotheses.
- Will closed or open models be more capable? If closed models are technically superior, will open models nevertheless be considered “superior” on a cost-adjusted basis? What is the trade-off between the quality of a generative AI response and its cost? How does this vary by vertical?
- How many of the potential uses of generative AI will result in new companies/applications, rather than new or improved functionality in the products of existing market leaders? Put another way, is the technology or distribution more important? Is there a hybrid model in which users access existing applications, such as PhotoShop or Microsoft Office, but while logging into a third-party AI service, such as OpenAI?
- Which AI products or integrations will warrant additional revenue from the user, rather than just be baked into the core product as a new table-stakes feature?
- To what extent are the answers to these questions path-dependent, that is, subject to specific decisions by specific companies and the quality of their specific products—as was the case with Meta open-sourcing its Llama 2 LLM). And how, again, do the answers differ by vertical?
Eventually, though, it will be necessary for parallel bets to be winnowed; all strategy is eventually about execution. Note how quickly Microsoft focused its OS strategy on Windows after the success of Windows 3.0 in 1990 (the company was later accused of following an “Embrace, Extend, Extinguish” model where one-time partners would be crushed once emerging markets stabilized). In April 2023, Google announced that its two core AI divisions, Google Brain (established in 2011) and Deep Mind (established with the 2014 acquisition of Deep Mind) would be merged into a singular unit, Google Deep Mind. The questions here, of course, are “When,” “How Much,” and “How do you know?”
Microsoft never halted its investments in applications and productivity tools, nor Internet services, and is better off as a result. Sometimes parallel bets lead to growth in new adjacent markets, rather than displace a current one (to that end, Microsoft’s more direct OS-bets were eventually paired). It’s possible that Amazon’s Alexa device footprint will still yet enable the company to regain market leadership. After all, the “era of AI” seems likely to involve some new device form factors, such as glasses (Amazon’s first pair of Alexa Frames debuted in 2018), and potentially pendants/badges, or new sorts of smartphones altogether (as OpenAI CEO Sam Altman and former Apple Chief Design Officer Jony Ive reportedly believe). As I detailed in the Streaming Book, there was nothing inherently wrong with Hollywood’s initial approach to internet-based video delivery, which spanned UltraViolet, IPTV VOD, TV Everywhere, à la carte SVOD subscriptions, licensing to SVOD services such as Netflix and Prime Video, distributing linear networks through virtual MVPDs such as Sling TV, and more. The issue was how long and hard Hollywood stuck to these experiments before properly embracing the “Streaming Wars” and the models proven by Netflix.
Of course, it’s not always clear when uncertainties have been “settled,” nor for how long. And there may be no field where this is more difficult to determine than in AI. In fact, the field’s pioneers have spent three quarters of a century debating what, exactly, “artificial intelligence” is and is not. In the 1950s, Marvin Minsky, a computer scientist who also co-founded MIT’s Artificial Intelligence Laboratory (and is fictionally attributed with the breakthroughs that enabled the creation of HAL 9000 in 2001: A Space Odyssey), offered one of the first commonly used definitions of AI—“the science of making machines do things that would require intelligence if done by humans.” Decades later, many AI researchers consider many anthropocentric definitions of AI to be misleading at best and unhelpful at worst as they suggest that intelligence requires a machine to process information like—if not outright behave similar to—a human. Still, the field has nevertheless aligned on an anthropocentric observation known as the “AI Effect.”
The “AI Effect” was coined by AI pioneer John McCarthy (who was, incidentally, Minsky’s co-founder at MIT’s AI Lab) in the 1960s to describe the “common public misperception, that as soon as AI successfully solves a problem, that solution method is no longer within the domain of AI.” Put another way, society quickly progresses from amazement at “artificial intelligence” to considering it not “real” Intelligence but instead just computation or, worse still, probabilities. A few decades later, Larry Tesler, who pioneered human-computer interfaces at Xerox PARC in the early 1970s and Apple in the 1980s, developed “Tesler’s Theorem,” which posited simply that artificial intelligence is “whatever hasn’t been done yet.” It’s through the AI Effect and Tesler’s Theorem that the one-time marvels of spell check—or an even more important AI benchmark, AI chess programs—have become so rote and superficially unimpressive. Someday soon transformer models may succumb to the same fate. To that end, OpenAI’s CEO Sam Altman has said that ten years ago “a lot of people” would have said “something like GPT-4” would be artificial general intelligence, but “now, people are like, ‘well it’s like a nice little chatbot or whatever.’”
What, then, is the future of today’s transformer models? In 2024, this architecture seems paramount. Their rate of improvement—which spans many different use cases, instantiations, and companies—has been too significant since this architecture was first described in 2017. And yet, this is the exact phenomenon that might lead various parties astray. Perhaps GPT-4 will be remembered not just as a “nice little chatbot,” but the wrong technical foundation overall. Especially for AGI.
Consider, as an example, the perspective of John Carmack, who is considered the “father of 3D graphics” due to his pioneering work at id Software, which he co-founded in 1991, and in 2013 joined Oculus VR as its first CTO. In 2022, Carmack left Meta to found Keen Technologies, a startup focused exclusively on creating artificial general intelligence (AGI), after which he explained to Dallas Innovates that he believed the number of “billion-dollar off-ramps” for AI technologies has become a de facto obstacle to achieving true AGI: “There are extremely powerful things possible now in the narrow machine-learning stuff . . . [but] it’s not clear those are the necessary steps to get all the way to artificial general intelligence. . . . Most of what the mainstream is doing [this research and development is] because it’s fabulous, it’s useful . . . [but] there is this kind of groupthink . . . that is really clear, if you look at it, about all these brilliant researchers—they all have similar backgrounds, and they’re all kind of swimming in the same direction.” It is not difficult to understand what Carmack is referring to when he refers to groupthink. The 2017 Google Brain research paper that proposed the modern transformer model is now the basis for OpenAI’s models (one of the paper’s eight authors joined OpenAI in 2019) as well as those of Anthropic, Cohere (founded by another author), Character.AI (founded by another author), Mistral (co-founded by an 8-year alumnus of Meta’s AI Research Lab), Inflection.AI (founded by a founder of Google DeepMind), Perplexity.ai (founded by an alumnus of Google DeepMind and OpenAI), and Meta’s LLaMA. Perhaps, then, Microsoft’s parallel bets are, for the most part, just different versions of the same bet?
For that matter, Meta’s Chief AI Scientist, Yann LeCunn has consistently argued that LLM and GPT models will never reach human intelligence levels, let alone AGI. In a May 2024 interview, he explained to Financial Times these models have “very limited understanding of logic . . . do not understand the physical world, do not have persistent memory, cannot reason in any reasonable definition of the term and cannot plan . . . hierarchically” and are “intrinsically unsafe” as they can operate accurately only if fed accurate information. A day later, he tweeted that “If you are a student interested in building the next generation of AI systems, don’t work on LLMs.”
In contrast to today’s consensus approaches, Carmack is optimistic about “a handful of ideas that are not the mainstream . . . some work from like the ’70s, ’80s, and ’90s that I actually think might be interesting, because a lot of things happened back then that didn’t pan out, just because they didn’t have enough scale. They were trying to do this on one-megahertz computers, not clusters of GPUs. . . . One of the things I say—and some people don’t like it,” Carmack continued, “is that the source code, the computer programming necessary for artificial general intelligence, is going to be a few tens of thousands of lines of code. Now, a big program is millions of lines of code—the Chrome browser is like 20 to 30 million lines of code. Elon just mentioned that Twitter runs on like 20 million lines of Scala. These are big programs, and there’s no chance that one person can go and rewrite that. You literally cannot type enough in your remaining life to write all of that code. But it’s my belief that I can really back up that the programming for AGI is going to be something that one person could write.” Would that make it a big bet or a small one?
Matthew Ball is the CEO of Epyllion. The fully revised and updated edition of his instant nationally and internationally bestselling book The Metaverse: Building the Spatial Internet will be released in July 2024. A version of this article first appeared on Matthew Ball’s website.